Image quality and video resolution are important but also complex parameters to determine in an end-to-end video workflow. This article will give an overview of the factors influencing these quality characteristics and will look at important process steps from imaging to the display device. The focus will be on how to unify the view of image quality, in the areas of image acquisition, post-production and distribution, in order to provide a better customer experience. Furthermore, a new image content-independent measurement method for video resolution determination is presented, which does not require a measurement target.
Picture quality
When talking about video resolution, the line counts 576p, 720p, 1080p and 2160p are often used to denote a particular video resolution. However, there is a big difference between the pixel count, which is derived from the aspect ratio and the number of lines, and the actual video resolution. Video resolution indicates the highest frequency that can be transmitted with an image signal. Even today, line pairs per image width or height are used to determine resolution. Here, a test signal is sampled and then the MTF (Modulation Transfer Function) is determined.
If digitally generated test signals are used to determine the performance of a film scanner or a camera, the effort is considerable. A suitable test target must be available and, in the best case, an automatic evaluation of the test results must be carried out. Most film scanners use area sensors. With digital cameras, the Bayer pattern has become established in different variants, in which only one colour information is sampled per pixel. The missing two colours are then interpolated from the surrounding pixels in the camera or in post-production.
This interpolation of colour values brings with it an inaccuracy, the size of which is essentially determined by the noise components created during the recording and the de-bayering algorithm used. Area sensors, on the other hand, have an image quality advantage because no interpolation is necessary here. If the image signal is recorded in an uncompressed RAW format and if these RAW data are correctly denoised before de-bayering, not only an image signal with optimal noise behaviour is obtained, but also a considerably higher image resolution, which results from the then optimal interpolation process of the de-bayering. The inaccuracies caused by noise during interpolation can thus be reduced to a minimum. The use of lossy RAW formats is very low for patent reasons, even if this patent allocation is questioned from time to time
The highest image quality and resolution results from uncompressed RAW, e.g. as a DNG sequence in a combination with high-quality RAW video denoising outside the camera (e.g. 9x7 camera Pawel Achtel and IRIS.RAW). If the (RAW) camera signal is lossy compressed, the noise components are modulated and a shift of frequencies into other frequency ranges occurs. Of course, denoising processes are also used in cameras. However, for performance reasons of the camera platforms, among other things, they do not come close to the image quality of the methods used outside the cameras. A very poor method in terms of image resolution is to permanently superimpose grain components on the image signal in the camera in order to give the DP the option of prescribing a fixed film look. In this way, any denoising artefacts can be covered up with the help of the artificially created grain components. As a result, however, the image resolution and the dynamic range are negatively affected in the long term.
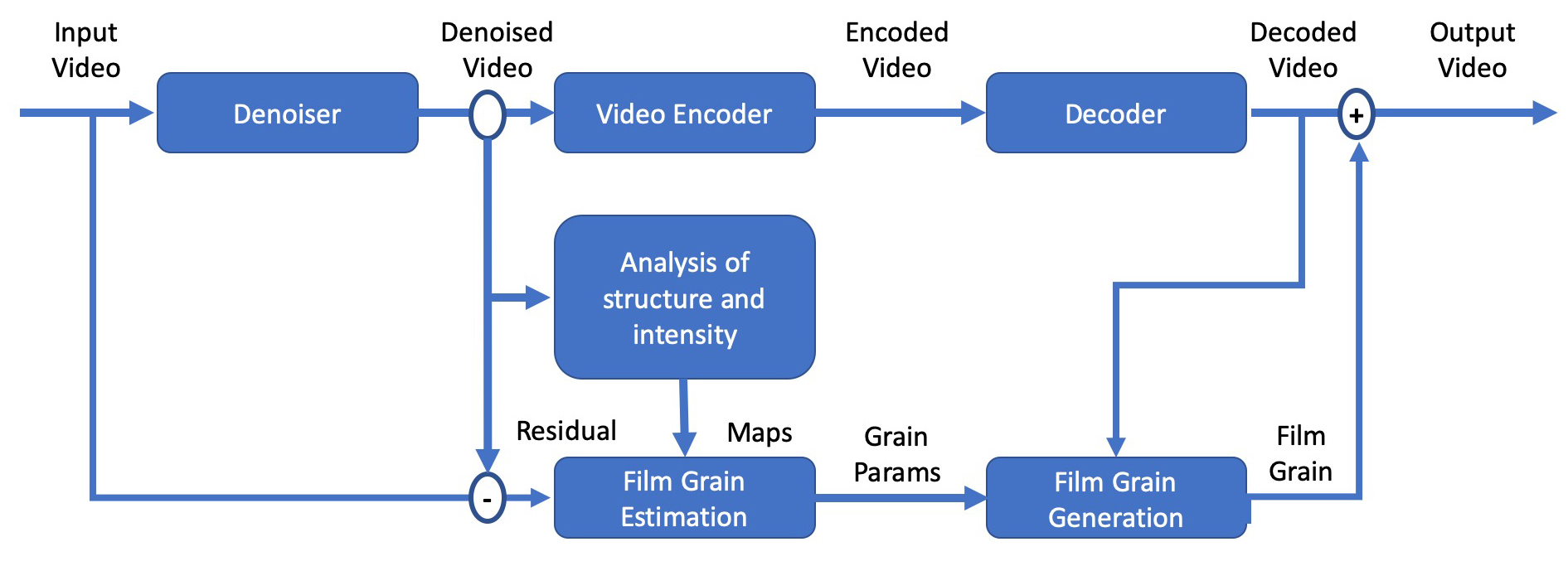
The effects of noise on video encoding
Video noise and grain in distribution result in degraded video quality, reduced video resolution and increased bandwidth requirements. Video codecs detect and encode superfluous information. The higher the compression, the more of the less important information is removed. Noise in the video content reduces temporal redundancy and forces the encoder to remove more original content. The encoder's ability to detect motion in a video sequence and correctly define the motion vectors can be greatly affected by noise. The accuracy of the motion vectors is essential for a high quality video encoding process. When noise is present, the encoder is forced to interpret the noise as motion, which results in erroneous motion vectors and is the reason for the increased bandwidth requirements of noisy video signals.
If the intra/inter decision mechanism detects that the motion estimation is too weak due to the given noise level, only intra prediction is used. A loss of inter-prediction will result in a loss of quality at the appropriate points in the video.Applying high-fidelity video noise management before the encoding process results in higher video resolution, better picture quality and optimised video bitrates. Even films with high grain content do not lose their film look if the denoising process is capable of doing so. However, this is not the case for all video noise management solutions available on the market.
If a grain structure similar to celluloid film is still recognisable in post, all that remains of the grain in the final device is a low-frequency noise signal, banding and blocking artefacts. Only at unusually high bit rates in distribution would this effect improve slightly without Video Noise Management. Independent of the encoder technology, it therefore makes sense to provide for the transmission of noise and grain components via a metadata model for all distribution channels. A corresponding specification is in preparation. AV1 is the first video encoder technology in which "film grain synthesis" is mandatory in the decoder. In the reference implementation (libaom) but also in other AV1 encoders, video denoising is already implemented as pre-processing before the encoder. However, the quality of this denoising is often not sufficient for professional applications. In this case, the film material is denoised before video encoding and the grain is only added again via parameters after decoding.
With "Film Grain Synthesis" (Fig. 1) it is possible that a film look can be created for broadcast and OTT that goes far beyond what can be experienced on screens today. Again, the denoising used should deliver high quality results and there is a danger that added noise and grain should only mask low image resolution or artefacts of the denoising. The great advantage of separating image content and noise components is that up to 30 percent bit rate savings can be achieved in distribution. Cost advantages in distribution, but also better streaming capability can be achieved in this way. The use of "film grain synthesis" already in the camera signal or in the intermediate format would lead to a considerably higher picture quality in distribution. The task here is to adapt the already existing specifications for the different workflow steps and to establish a comprehensive understanding of this problem.
Video resolution
The video resolution is not bound to a number of lines, but can vary from frame to frame or scene to scene. In only a few cases does it reach the maximum possible resolution resulting from the number of pixels and the aspect ratio. The respective video camera used and the quality of the lenses form the basis of what maximum video resolution can be achieved. The video resolution is further determined by a missing or insufficient video noise management and the selected lossy video encoding formats for acquisition and post-production. Each additional lossy re-encoding step can have a lasting impact on video resolution. Caution is also advised with the technical term "Visually Lossless Compression". Even if no significant image degradation can be perceived without measurement technology in the image acquisition, the noise components are transformed into other frequency ranges. The effects on the picture quality then arise in the post-production or distribution of the video signals. The noise components can then only be used to a limited extent for noise analysis of the video signal and reduce the quality of the video noise management results. If no video noise management takes place in post-production, the lost headroom of the noise components cannot be used for additional contrast and HDR optimisation.
Picture quality measurement methods such as PSNR, VMAF or SSIM are used in distribution to compare the intermediate format with the distribution format. At the bit rates intended for distribution, the picture signal is already so highly compressed that measurement methods such as PSNR provide only limited useful results. VMAF was initially developed by Netflix to find the optimal bit rate for each content (per title/per shot encoding). High motion, grain and noise content in video leads to high bandwidth requirements, while digitally generated content without noise leads to low bandwidth requirements. VMAF still offers quality gradations even at high compression rates, but it can still only be used for encoder comparisons to a limited extent. The VMAF measurement results can be influenced by manipulating the image signal in the encoding process. Work is certainly being done on a more robust VMAF version, but manipulation of the results can currently only be ruled out to a limited extent. Further work is being done to find a solution for how VMAF can be used when using "Film Grain Synthesis".
For quality assessments, the intermediate format is used as a reference. However, the picture resolution of the intermediate format, which is independent of the number of lines, is not included in the measurement result. When assessing the VMAF values, it is therefore not known for which video resolution the measurement results apply. Measurement results of the measurement method for "image content-independent measurement of video resolutions" presented in the following show that the image resolution varies between 60 per cent and 100 per cent of the maximum resolution possible by the number of lines. Furthermore, in a VMAF evaluation it is not known which video resolution the video test material has. A reference material with a significantly reduced video resolution means that the tested encoders only have to process a reduced frequency bandwidth at the input. This tends to produce VMAF results that are too good, since the encoder with a reduced bandwidth at the input delivers better encoding results. If a post-production line is then able to produce an intermediate format with higher video resolutions, the quality gains could be penalised with poorer VMAF values, as the encoders used could deliver poorer results with the now increased frequency bandwidth at the input.
Image content-independent measurement method for video resolution determination
The introduction of measurement targets into the image signal is costly and also does not reflect the video resolutions that can be achieved in everyday life. A new image content-independent measurement method for video resolution determination, which can be integrated into the image signal chain, was developed by the company Wavelet Beam and will be presented in the following. The measurement procedure can be applied to RAW video data as well as to already de-bayered video material (Fig. 2: Pos 2). In a further step, the video signal is subjected to a transformation (Fig. 2: Pos 3) into the frequency or time-frequency domain. Since the video resolution can be different in the colour channels, the transformation should be done per colour channel. Then the coefficients are analysed (picture 2: Pos 4) and sorted according to frequency components. In a fifth step (Fig. 2: Pos 5), the upper cut-off frequency is then determined using, for example, statistical methods. The horizontal and vertical number of pixels defines the maximum video resolution. An additional normalisation (Fig. 2: Pos 6) of the measurement results enables the use of the measurement method at different points in the workflow.
An important unique selling point is that the measurement method is independent of image content. This means that even a very small number of high-frequency image components is sufficient to determine the image resolution. A target in the image is not necessary and thus enables continuous monitoring of the image signal in production. In image acquisition, this new method can be used to assess the achieved image sharpness for each camera position or to support automatic QA of external video material. In distribution, on the other hand, this procedure can be used to select test clips. In this way, there is no longer the danger that the measurement results of picture quality assessment procedures such as VMAF, SSIM or PSNR reflect too low a picture resolution. This in turn, of course, also has an impact on the assessments of the VMAF or PSNR values, because these can now be related to the actual video resolution. A VMAF evaluation could therefore look like this in the future: Encoder A has a picture quality that is 1.5 VMAF points better than encoder B at a bit rate of 13200 Kbit/s with an average video resolution of 3.24K horizontal (1.63K vertical).
This is important because in practice recommendations are sometimes made that the intermediate video material can be reduced even further in bit rate, as the VMAF measurement results would only provide a slight change to the lower compressed video material during distribution. Such a statement is highly critical, as the VMAF difference values should refer to the real and measured image resolution and not only to the number of pixels in the image. If an intermediate format is subjected to too much compression, resolution is also lost and a further reduction of the bit rate of the intermediate format only results in a small VMAF difference. But this is really only the case with a then significantly reduced image resolution. Unfortunately, almost all VMAF evaluations lack the indication of the measurement error and thus the possibility to also correctly evaluate the VMAF results..
End Devices
End devices such as UHD televisions and high-resolution second screen devices are already widespread in Germany. However, the possible picture quality is further reduced by the IP infrastructure in many flats and houses as well as by further picture processing steps after decoding the picture signal. In the case of television sets, the default values selected by the manufacturers for colour, sharpness and contrast are often far too high. Furthermore, the denoising and de-blocking is preset as default and often has only a very low quality. In principle, however, it must also be stated that denoising at the end of the distribution chain after the decoder is the worst of all options. If noisy picture signals are encoded for distribution, they require a much higher bit rate to achieve a comparable quality impression in contrast to low-noise signals. The reduction in the required bit rate of up to 30 percent stated by Netflix, which is possible through video noise management, could be confirmed in practice with Wavelet Beam.
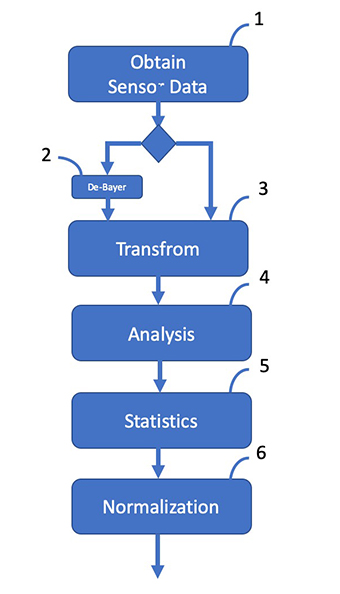
The user needs a certain amount of expertise to optimally adjust a television. The "Filmmaker Mode "8), which can deactivate some of the default settings via only one menu item or one button on the remote control, is a help here. An automatic mode that can automatically activate or deactivate the "Filmmaker Mode" per programme on the basis of the genre or a DVB descriptor would be very desirable. With a dynamic film maker mode, sports programmes could continue to benefit from the higher frame rates, but feature films intended for playback at 24 fps could also avoid the so-called soap opera effect. Since televisions and second screen devices are often connected via WLAN, there is a risk that the distance between repeater or router is too great and only a very limited bit rate is available. A WLAN bit rate that is a few percentage points too low can make the difference as to whether a programme can be received in maximum UHD or only in 1080p. For broadcast services, this danger does not exist in principle, but it must first be proven what picture quality the encoder links actually deliver. Live video encoding currently offers much poorer encoding efficiency than offline encoding for OTT. Currently, the picture quality for OTT is even higher than that of broadcast channels. A higher encoding time for offline encoding is decisive for the encoding efficiency. Since OTT services are often consumed on second screen devices and the distance between the screen and the viewer therefore falls below 70 cm, this higher video quality can also be perceived by the viewer. The smaller the viewing distance, the higher the possible perceptible video resolution. In addition to this, there is only a slight manipulation of the image signal on second screen devices. Here, no black and contrast values are changed, but real-time video denoising procedures are not likely to be found on second-screen devices, and thus a further reduction in video resolution on OTT need not be expected. DVB broadcast competes with OTT offerings in terms of broadcast content and picture quality. A large proportion of young people in Germany use OTT services almost exclusively, against which the broadcast sector must be measured. Only a very small part of broadcast content is actually live. Therefore, a large part of the programme can already be homogenised today through highly efficient HPC video noise management solutions.
Figure 2: Wavelet Beam: An image content-independent measurement method for video resolution determination
A reduction of the noise components then ensures that the picture quality does not drop in demanding scenes. Of course, video noise management should not simply smooth the picture signal and thus destroy the film look. "Film Grain Synthesis" as in AV1 already offers the availability of an end-to-end distribution chain for all AV1-capable end devices. Even without "Film Grain Synthesis", a high-quality picture impression should be guaranteed. For 24/7 broadcast channels, performance but also power requirements are very important. Load balancing between CPU and GPU is the key technology here. Only if several files can be processed simultaneously on one GPU and several GPUs are used per server, the required hardware can be used efficiently.
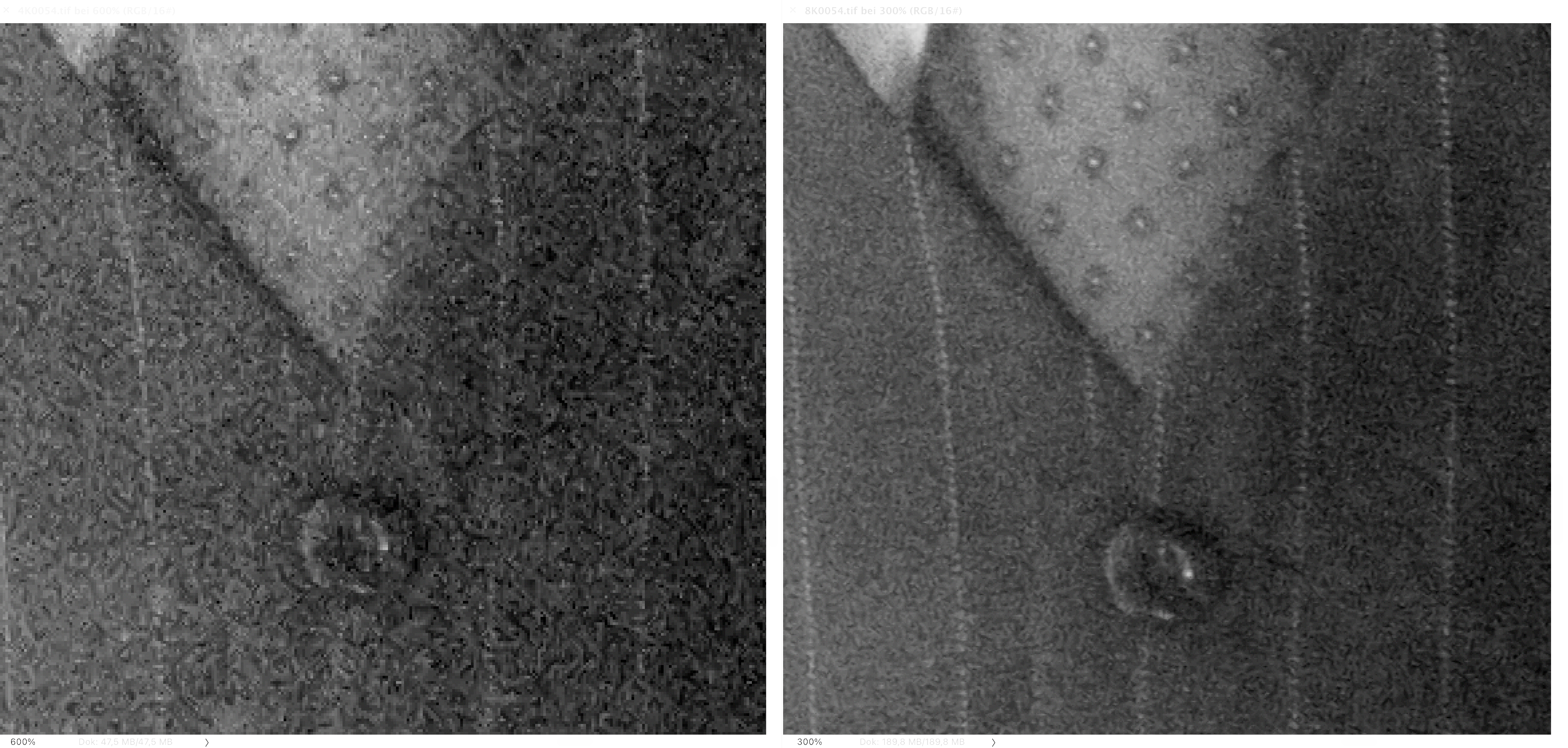
AI based Video Up-Scaling
Traditional video upscaling filters (Bicubic or Lanczos) use interpolation techniques to increase the image size by extrapolating existing pixel values and filling the gaps between pixels. This method can result in a loss of image quality and detail because it does not include information about the actual image. In contrast, AI-based video upscaling uses machine learning to develop a deep understanding of the underlying image and generate the missing pixels based on this knowledge. This is achieved by using large data sets of high-quality images and videos to train a neural network that is able to recognise complex patterns and upscale the image accordingly. Through this method, the AI can make more accurate predictions and achieve higher image quality and detail than traditional upscaling filters. In addition, the AI can also identify specific features of a video or scene and enhance them accordingly to achieve a better overall image. Overall, Kl-based video upscaling offers a higher level of precision and quality than conventional methods.
It is important to perform video denoising before AI-based upscaling, as noise in a video can make it difficult for upscaling algorithms to produce high-quality results. If a video contains noise, the upscaling algorithm may misinterpret the noise as actual image detail. Removing the noise from the video before upscaling can provide the upscaling algorithm with a clean and high-quality video signal, resulting in better upscaling results and better visual quality of the upscaled video. When combining AI-based upscaling and video noise management, super-resolution effects can occur, which can be impressively seen in Figure 3.
Summary
The video resolution of the intermediate format used to generate the distribution encodes is usually not known. Often, the actual video resolution is more than 30 per cent lower than that which would be calculated from the number of pixels and the aspect ratio. The video resolution determination procedure presented above can be used to select demo material, to support "per title/shot encoding" or to determine the focus point of a camera setting. Furthermore, it is helpful wherever viewing distances and their influence on the perception of e.g. 4K/8K HDR video quality are to be scientifically investigated. It was shown which different factors can influence the image quality in the end-to-end workflow. The lack of specification of the video resolution and error tolerance in the image quality assessment makes it easy for many marketing departments to differentiate their products from the competition through avoidable performance features.
A quote from Steve Yedlin fits this very well:
„The dominant narrative is that as long as we know this one number. We don‘t need to know anything else about the complex pipeline that defines our master image, because that one number, we believe somehow corresponds to an audience‘s perceptual experience of sharpness and clarity.”
A good broadcast and VOD product is characterised by many factors, but good audio and video quality should always be the goal and serve as a quality feature. Not only "because we can", but also because it is economically very attractive.
- Pawel Achtel, 9x7 Camera
- Wavelet Beam, RAW Video Denoising
- Andrey Norkin and Neil Birkbeck, Film Grain Synthesis for AVl Video Codec
- Andrey Norkin & Liwei Guo from Netflix, DEMUXED 2019
- Zhi Li, Netflix, On VMAF‘s property in the presence of image enhancement operations
- Wavelet Beam, Copyright Q2/2023, Image content-independent measurement method for video resolution determination
- HDTVTest, TV Settings That Should be ILLEGAL
- FILMMAKER MODE